Snowflake has swiftly risen to prominence in the data world, and its consumption-based pricing model is well-known among data professionals. However, it’s time to clear the air: Snowflake isn’t out to empty your pockets. In fact, there is a suite of tools and features to help you with Snowflake cost optimization and align spending with your actual requirements.
No one wins with wasteful spending. Instead of burning through credits on inefficient SQL queries, Snowflake would rather you channel those resources into higher-impact projects. These not only advance your business objectives but also enhance the stickiness of Snowflake’s solution.
It’s a symbiotic relationship: as businesses thrive with high-value projects, Snowflake sees a more sustainable and organic growth in long-term consumption.
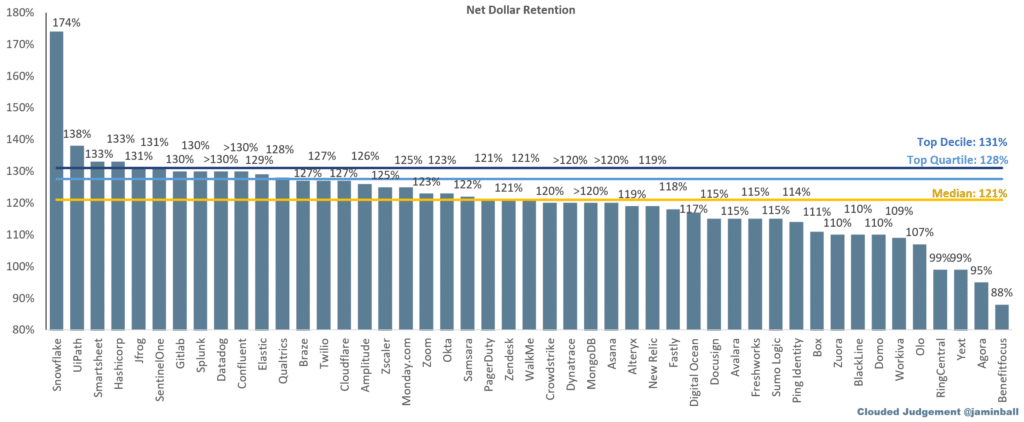
Source: Clouded Judgement. Snowflake’s high net revenue retention demonstrates the value the platform brings to its customers.
While Snowflake’s native tools and recommendations are undeniably valuable, they sometimes might not capture the full breadth of optimization possibilities available to data teams.
So what’s the next step after maximizing Snowflake’s native capabilities? That’s what we’re here to discuss: Snowflake cost optimization. We want you to grasp your Snowflake spending fully, so you can keep it in check, optimizing further and deriving the best value from every credit.
The Nuances of Snowflake Costing
Snowflake’s pricing strategy is an exemplification of its user-centric approach: pay for what you use. But what does this really mean in practice, and how does it shape the strategies for cost optimization?
Usage-Based Pricing
At the heart of Snowflake’s costing is its usage-based model. Unlike flat-rate structures where you might end up paying for resources you never utilize, Snowflake ensures you’re only billed for your actual consumption. This offers companies a unique advantage — costs can be tightly monitored, they are instantly adaptable to more efficient management, and most importantly, they can be precisely aligned with business value, making ROI calculations transparent.
Breaking Down Snowflake Costs
While the underlying philosophy is straightforward, the actual computation might seem intricate due to various factors at play. Here’s a simplistic breakdown:
- Storage: How much data is stored in Snowflake.
- Compute: Resources consumed for processing and querying the data.
- Cloud Services: Operations related to infrastructure management, like authentication and coordination.
- Serverless Features: These are additional functionalities Snowflake offers that can be tapped into as needed.
Most Snowflake users transact using Snowflake credits, essentially a currency that offsets consumption costs. These credits are available on-demand, though one can opt for pre-purchasing capacity for foreseeable requirements. To give you a snapshot, as of October 2023, in the AWS-US West region, the on-demand storage pricing stood at $40 per terabyte per month. Depending on the selected plan (Standard, Enterprise, Business Critical), Snowflake credits ranged from $2.00 to $4.00.

Example Snowflake pricing in the AWS – US West region. Source: Snowflake Pricing.
Translating your regular work operations into Snowflake credits might seem daunting, but many third-party platforms offer calculators (see here and here), and Snowflake’s own pricing guide is replete with examples.
The essence here is to understand that your Snowflake costs predominantly revolve around credit consumption, which in turn hinges on storage and compute. The more tables you have, the kind of SQL queries you run, and the dimensions of your data warehouses are pivotal determinants.
So Why Do Snowflake Costs Become Prohibitive?
When looking into Snowflake cost optimization, grasping the intricacies of Snowflake’s costing mechanisms is pivotal for any data professional. On the surface, it appears transparent and direct: costs emerge from credit consumption, which is influenced by storage, compute, and the nature of operations.
Yet, despite this apparent straightforwardness, many organizations find themselves startled by ballooning Snowflake expenses. And users are vocal about it.

So, what’s the missing piece of the puzzle? Why do costs sometimes surge unexpectedly? Let’s delve deeper into the underlying reasons that can drive these unforeseen spikes in your Snowflake bill.
1. Complexity Beyond Simple Computation
It’s a misconception to assume that Snowflake costs directly correlate with computation time. While compute duration does play a role, it’s intertwined with numerous other factors. The type of query, data volume, and specific features or services utilized can all impact the final bill. It’s not just about how long the wheels are turning, but also about what kind of tasks they are performing.
2. Method Matters
In Snowflake, there’s often more than one way to accomplish a given task. Each method or approach can come with its own cost implications. For example, choosing between using a smaller warehouse for a more extended period or a larger one for a shorter span can result in different costs, even if the end result remains the same. This flexibility is both a boon and a challenge, demanding careful consideration of methods for optimal cost-efficiency.
3. Ad-Hoc Queries
Ad-hoc queries are often impromptu, one-off queries fired by business users to fetch specific data or insights. They are unpredictable, may not always be optimally written, and can vary widely in their resource consumption. However, they’re an essential aspect of dynamic business environments where real-time decision-making is required.
While ad-hoc queries can be resource-intensive, attempting to fine-tune each one isn’t always feasible or cost-effective. Given their unpredictable and one-off nature, the return on investment (ROI) for optimizing them is often low. Instead, it’s more pragmatic to focus on providing guidelines or best practices for business users to follow, ensuring that these queries are not entirely off the rails.
4. The Predictability of Pipelines
In stark contrast to ad-hoc queries, pipelines are where cost optimization efforts can yield significant dividends. Given their predictable and repetitive nature, even small efficiencies or improvements can compound over time, leading to substantial savings. By optimizing the structure, scheduling, and resource allocation of pipelines, businesses can control and reduce their Snowflake costs considerably.
While Snowflake offers comprehensive guidance on optimizing warehouse sizes, queries, and tables, among others, there’s a significant area they touch upon less: the optimization of data pipelines. These pipelines form the very backbone of how businesses process, analyze, and act on their data.
It’s no exaggeration to state that they frequently command the majority of resources and subsequently, a large chunk of expenses. Given their foundational role and resource-heavy nature, it’s imperative to scrutinize and refine them for cost efficiency. That’s precisely our focal point in the upcoming section.
Why the Engineering of Data Pipelines is Crucial for Snowflake Cost Optimization
Data pipelines ensure the seamless flow of data from source to storage, and from there to the applications and users who derive insights from it. When you’re working with Snowflake, or indeed any data platform, the way these pipelines are engineered can have a profound impact on costs. Let’s delve into why the construction and management of these pipelines are paramount for cost optimization.
1. The Pitfalls of Ad-Hoc Pipeline Construction
Building data pipelines on the fly or in response to immediate needs may provide a short-term solution, but it also paves the way for long-term challenges. Such ad-hoc approaches often lack foresight, standardization, and scalability. Over time, they can lead to:
- Fragmentation: Multiple pipelines doing similar tasks, causing redundancy.
- Maintenance Challenges: As needs evolve, these pipelines become harder to update or modify.
- Performance Issues: Without optimization, they can consume more resources than necessary.
All these challenges cumulatively result in technical debt — a hidden cost that organizations will eventually have to pay, either in terms of rework, increased maintenance, or higher resource consumption.
2. The Need for Expertise in Pipeline Refinement
Optimizing data pipelines isn’t merely about cutting down on resources; it’s an art that involves understanding data flow patterns, user needs, and the intricacies of the platform (in this case, Snowflake).
- Complex Decisions: Deciding on partitioning, determining optimal data formats, and setting up caching are all decisions that require deep knowledge.
- Iterative Process: Refinement isn’t a one-time task. As data volumes grow and business needs change, pipelines need regular revisiting and tweaking.
The depth and breadth of knowledge required mean that this isn’t a task just anyone can handle. It requires specialists with experience in Snowflake’s ecosystem and data engineering principles.
3. The Cost of a Dedicated Engineering Team
Having a dedicated team of experts can be invaluable. They can craft, refine, and maintain data pipelines, ensuring optimal performance. However, this expertise comes at a price:
- High Salaries: Data engineering experts, especially those familiar with platforms like Snowflake, command competitive salaries.
- Training Costs: The tech landscape is ever-evolving. To keep the team updated with the latest best practices and features, regular training is essential.
- Infrastructure Overheads: A dedicated team needs tools, software, and sometimes even dedicated infrastructure, adding to costs.
While the benefits of such a team are undeniable, for many businesses, especially smaller ones, the costs can be prohibitive.
4. The Appeal of Automated Solutions
Given the challenges and costs associated with building and maintaining data pipelines manually, many businesses are turning to automated solutions. Off-the-shelf automation tools offer:
- Rapid Deployment: Pre-built solutions can be quickly integrated, speeding up the pipeline setup.
- Best Practices: These tools are usually built on industry best practices, ensuring efficiency.
- Scalability: They can often handle growing data volumes without significant rework or intervention.
- Cost Savings: By eliminating the need for extensive manual work and reducing the risk of errors or inefficiencies, businesses can achieve cost savings.
In essence, data automation provides a pathway to harness the power of well-engineered data pipelines without the associated high costs or complexities.
The way data pipelines are engineered and managed can make or break a company’s data strategy, especially when it comes to cost. Whether through expert teams or automation, a thoughtful approach to data pipeline engineering is a foundational step toward cost-effective data operations in Snowflake.
The Power of Intelligent Data Pipelines in Snowflake Cost Optimization
In the realm of data management, the notion of “intelligent” has evolved beyond mere buzzwords. Today’s dynamic business environment demands not only flexibility but also the ability to make informed decisions rapidly.
When it comes to data pipelines, the traditional “set it and forget it” approach is no longer tenable. This is where intelligent data pipelines come into play, pioneering a paradigm shift in how businesses handle, process, and analyze their data, especially within the Snowflake ecosystem.
Understanding Intelligent Data Pipelines
The backbone of an intelligent system lies in its ability to make decisions based on data and evolving patterns. These decisions, especially in the world of Snowflake, directly correlate with costs.
- Diverse Savings Opportunities: While the potential to save costs using Snowflake exists, pinpointing those savings is not straightforward. It often requires a deep dive into various dimensions such as storage, compute, and usage patterns.
- Maximizing Value, Minimizing Spend: The ultimate goal of any cost optimization strategy is to derive more value without proportionally increasing expenditure. Intelligent data pipelines aim to maximize the efficiency of every byte of data and every second of compute.
- Holistic Automation: Rather than applying patches or isolated strategies, intelligent pipelines look at the entire data lifecycle. They then apply automated data pipeline strategies that are not just reactive but also proactive, anticipating needs and adjusting in real time.
Read More: What Are Intelligent Data Pipelines?
Features of Intelligent Data Pipelines
What sets Ascend’s offering apart is its features tailored for the intelligent consumption of resources.
- Incremental Processing: One of the major costs in any data operation is rerunning entire batches of data for minor updates. Ascend’s pipelines allow for incremental batches and partitions. This means only the changed data gets processed, reducing compute costs and ensuring fresher data.
- Zero Rerun Restarts: Traditional pipelines, when interrupted, often require a complete rerun, consuming time and resources. Ascend allows pipelines to be stopped and restarted from the exact point of interruption, ensuring no redundant computation.
- Advanced Partitioning: Data partitioning, when done right, can significantly speed up operations. Ascend’s pipelines come with a sophisticated partitioning strategy that is seamlessly integrated into its automation framework, ensuring optimal data organization and faster query times.
- Real-time Resource Monitoring: Keeping a tab on Snowflake resource usage can prevent unforeseen costs. Ascend’s pipelines offer real-time monitoring, allowing businesses to adjust operations based on current consumption.
- Quick Diagnostics: Time spent diagnosing issues in a data pipeline can lead to increased costs. Ascend’s ability to detect resource hotspots within minutes means quicker resolution and less downtime.
- Optimal Cloud Resource Utilization: Ascend’s pipelines can leverage low-cost cloud services. By intelligently using features like tiered storage and interruptible compute, they ensure that businesses get the best out of their cloud investment without compromising on performance.
In an age where data is voluminous and dynamic, traditional data pipelines can become bottlenecks both in terms of performance and costs. Ascend’s intelligent data pipelines, with its innovative features, are tailored for today’s data needs, ensuring businesses can make the most out of their Snowflake investment.
Value and Efficiency in Snowflake Cost Optimization
Managing Snowflake costs effectively isn’t just about minimizing expenses, but about maximizing the value behind each cost:
- Embrace Transparency: Link costs to specific data outputs. This clarity allows for informed investment decisions and showcases the value of data-driven initiatives.
- Utilize Automation and Intelligence: Elevate ROI by leveraging automation and intelligent tools. Solutions like Ascend’s intelligent data pipelines demonstrate how automation amplifies efficiency and cost savings.
- Strategic Cost Management: Avoid hasty, indiscriminate cost-cutting. Instead, make data-driven decisions that prioritize the organization’s long-term health and the continued value of its data products.
In essence, Snowflake cost optimization is about striking a balance: ensuring each dollar spent yields maximum value, propelling businesses forward.