Article updated on November 14, 2024.
With the scope of data engineering rapidly evolving, “data automation” stands as a beacon of hope for overworked data engineering teams, yet it remains a frequently misinterpreted term. Despite its growing popularity, it’s not uncommon for data professionals to confuse data automation with data orchestration or consider it a subset of analytics, data mesh, data integration, or DataOps.
Such misconceptions aren’t unfounded, given the far-reaching nature of data automation that encapsulates everything we do as data engineers — from ETL pipelines to data observability.
This article aims to shed light on this critical concept, delineate its differences from other related terms, and illustrate the profound advantages it offers to data and analytics engineering teams. Specifically, we’ll delve deep into what exactly is data automation, exploring its importance, benefits, working mechanisms, and the optimal tools to harness its full potential.
What Is Data Automation?
Data automation, at its essence, is the strategic utilization of technology to streamline and enhance data-related processes. Instead of getting bogged down by repetitive and time-consuming tasks, data professionals leverage automation tools to handle these routine operations efficiently. With data volume on the rise within modern organizations, automation enables teams to process and analyze vast datasets efficiently, ensuring data integrity and quality at scale.
The primary aim of data automation is not to replace human expertise but to augment it. Automating mundane and manual tasks frees up data professionals to channel their skills and intellect toward more impactful, high-value projects. This, in turn, empowers them to drive meaningful business outcomes, foster innovation, and harness the potential of data in advancing organizational goals.
Differences Between Automating Data and Orchestrating Data
While the terms “automating data” and “orchestrating data” often find themselves used interchangeably in data engineering discussions, they serve distinct roles in the data lifecycle. Understanding the nuances between the two is vital for data professionals aiming to optimize their workflows and derive the most value from their data operations.
Purpose and Scope:
- Automating Data: Data automation primarily focuses on leveraging technology to handle repetitive data processes without constant human intervention. It aims to eliminate manual, routine tasks, allowing data professionals to concentrate on more strategic activities that can directly benefit the business.
- Orchestrating Data: Data orchestration refers to the coordinated management and execution of different data-related tasks in a specific sequence or flow. It’s about ensuring that multiple data processes, which could be automated or manual, interact seamlessly and execute in the right order for a desired outcome.
Human Involvement:
- Automating Data: The main objective is to reduce the need for continuous human intervention in routine tasks, providing professionals with more time for in-depth analysis, strategy development, and high-level decision-making.
- Orchestrating Data: While it may involve some automated processes, orchestration often requires human oversight to ensure that data tasks are coordinated effectively and that dependencies between tasks are managed properly.
Outcome:
- Automating Data: The primary outcome of automation is efficiency — performing a specific data task faster and with minimal errors.
- Orchestrating Data: The outcome here is coherence — ensuring that all data tasks, whether automated or not, culminate in a harmonized, coordinated result that aligns with the overall data strategy.
In summary, while both data automation and orchestration are critical to modern data operations, they cater to different aspects of the data lifecycle. Data orchestration is primarily about choreographing specific tasks based on set parameters. These tasks are initiated by various means, be it timers, triggers, or dependencies within a DAG (Directed Acyclic Graph).
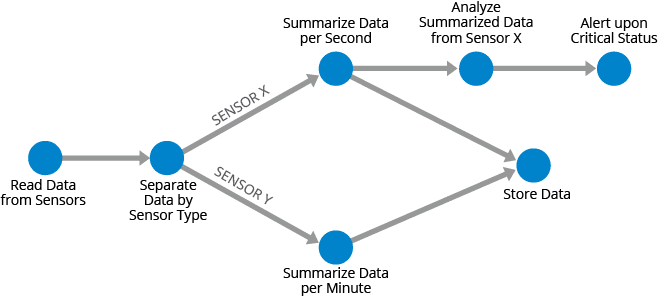
In contrast, data automation operates fundamentally on a metadata-driven framework. Data automation systems amass extensive metadata concerning the data they oversee, encompassing detailed data models, historical patterns, frequency of data changes, and a clear lineage of data’s origin and destinations. This vast metadata pool is then leveraged to guide and refine the actions of the system, enhancing the entire data engineering process.
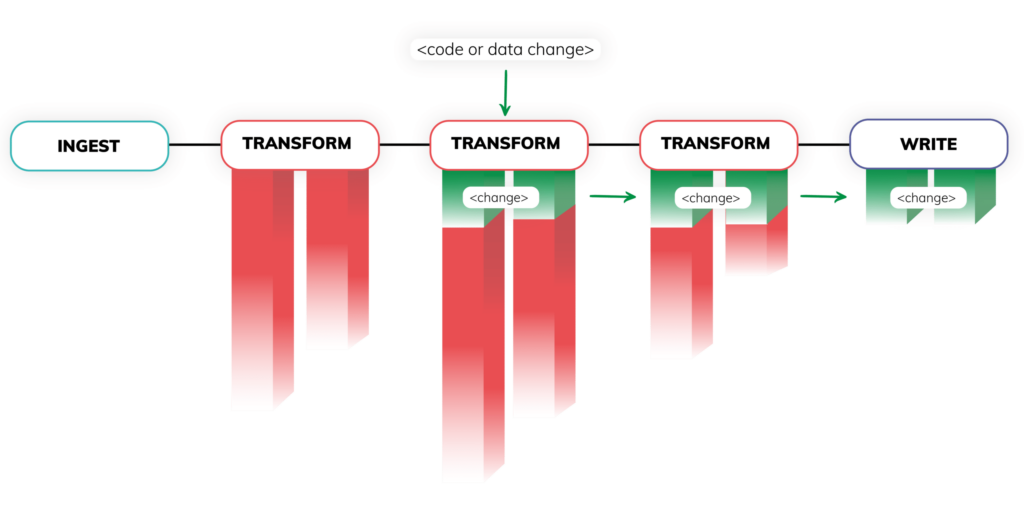
How Does Data Automation Work?
Still sounding a bit confusing? Data automation becomes clearer when put into the context of ETL (or ELT, or to be more precise, EtLT). Throughout the process, metadata is the common denominator enabling automation at each step.
Step 1: Extract
Advanced data automation strategies offer capabilities that go beyond basic extraction. They maintain historical records of prior extractions, understand the data’s profile, track its change frequency, and are mindful of the system’s load parameters from which data is sourced. Why is this extensive metadata crucial? It helps the automation system answer a myriad of critical questions. For instance:
- Should data reading be restricted to a few threads to avoid overwhelming a small database?
- Or is it an object store where you can parallelize reads across hundreds if not thousands of workers all at the same time?
- Has this specific data been extracted before? If so, has it changed and does it need to be re-extracted?
By addressing these questions, automation can do the work of understanding the nature of the other parts of our data ecosystem and how it all works together.
Step 2: Transform
When it comes to data transformation, automation goes beyond the notion of orchestration. It aims to enhance both the movement and processing of data. Data automation processes retain a memory of the historical resources used, the meticulous lineage of data as it weaves through systems, and the access patterns of resulting data sets.
With this context, data automation is able to ensure jobs are more efficient and less prone to error by automatically answering questions such as:
- Do we need to even run a new data processing job? If so, how many resources should it have?
- Does it run better on a particular type of engine than another?
- What depends on this job and others that are competing for resources, and based on those dependencies, is one higher priority than the others?
Step 3: Load
Until recently, few companies automated this step, but that’s starting to change — and the introduction of “Reverse ETL” as a category should help accelerate innovation. Today, companies are using automation to answer questions such as:
- What if data already exists where I want to load new data?
- Is the data correct and does it have the right schema?
- What do I do when the schemas don’t match?
Data automation, fueled by the volumes of metadata collected, enables us to efficiently and accurately answer these questions. This not only minimizes the strain on downstream systems but also expedites the data delivery process, ensuring efficiency and precision.
Read More: Moving Past ETL and ELT: Understanding the EtLT Approach
Developing a Data Automation Strategy
In the context of data automation, various elements shape a successful strategy, many of which are unique to each company’s circumstances and objectives. However, one universal constant emerges as indispensable: the transition from the traditional imperative approaches, characterized by rigid, hard-coded rules, towards more adaptive declarative methodologies.
Understanding Imperative Approaches:
Imperative workflows are deeply rooted in data orchestration systems. These workflows prescribe a step-by-step guide to achieve a goal: classify data, outline transformations, develop the ETL process, and so on. While this might offer a perceived sense of control, it makes processes vulnerable. Rigid rules and manual operations in imperative methods make the system inflexible, slow to adapt to changes, and often result in prolonged development cycles. Such workflows, while sequential and well-defined, lack the agility required for rapidly changing data ecosystems.
Embracing the Declarative Paradigm:
Contrastingly, a declarative approach paints a picture of the desired outcome, without explicitly listing down every action required to achieve it. Here, the focus shifts to the ‘what’ rather than the ‘how’. For instance, rather than manually scripting every data movement, a declarative system is provided with the end goal, and it autonomously determines the best route to achieve it. This self-governing ability comes from its deep reliance on metadata.
With a declarative approach, data engineers can set objectives, and the system intelligently designs the process. It integrates seamlessly with existing developer tools, harnessing data automation’s full potential, from data integrity checks to smart rollbacks.
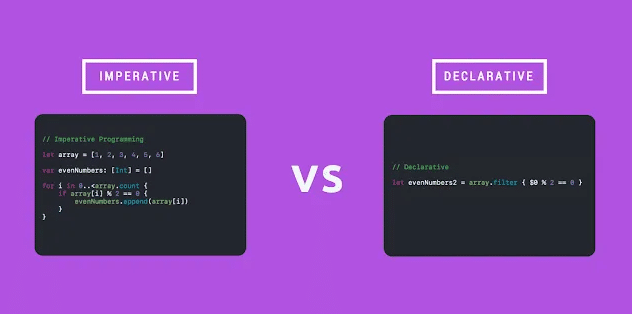
The Role of Metadata in Declarative Systems:
The backbone of a declarative system is the wealth of metadata it harnesses. This vast repository captures vital details, such as data profiles, access patterns, and resource requirements, to name a few. Gathering this metadata might pose challenges initially, but its rewards are manifold. It’s what empowers the system to be dynamic, adaptive, and intelligent.
In conclusion, developing a data automation strategy for the modern age means prioritizing flexibility and adaptability. Transitioning from an imperative to a declarative approach is not just a technological shift; it’s a mindset change. It encourages businesses to look beyond the immediate, manual operations and embrace the future’s expansive, autonomous possibilities.
Whitepaper: Workflow Automation Systems Vs. Dataflow Automation Systems
The Benefits of Data Automation
The significance of automating data processes cannot be overstated. Below are the multifaceted advantages that data automation offers:
Time Savings:
- Overview: Automating routine tasks offers organizations the opportunity to save crucial hours that can be otherwise used for more complex and strategic activities.
- Impact: Freeing up staff from monotonous and time-consuming tasks allows them to channel their focus towards areas that require human creativity, fostering overall efficiency. This can speed up decision-making processes and reduce the time taken to achieve critical milestones.
Enhanced Performance:
- Overview: Automation streamlines operations, eliminating potential bottlenecks and inefficiencies.
- Impact: By expediting outputs and ensuring swift response times, businesses can ensure that their data systems remain agile and responsive. This quick turnaround can significantly improve customer experiences and operational workflows, keeping businesses a step ahead of the competition.
Scalability:
- Overview: Data automation is designed to be adaptable, and capable of expanding its operations in line with growing data requirements.
- Impact: As organizations grow and their data loads surge, the flexibility of data automation means they can scale up (or down) without the need for major system overhauls. This ensures continuity of operations and the ability to seize new opportunities without technological limitations.
Cost Efficiency:
- Overview: Automation reduces the dependence on labor-intensive manual processes.
- Impact: With fewer manual processes, companies can significantly curtail overhead costs related to human resources, error corrections, and process delays. Over time, the cost savings from automation can be channeled into other strategic investments, bolstering a company’s competitive edge.
Improved Data Quality:
- Overview: Automation, by design, is consistent, following the same process every time without deviation.
- Impact: This consistency minimizes the risk associated with human errors such as data duplication, misentry, or omission. As a result, the data is of a higher quality, more reliable, and more accurate. Reliable data is the foundation of informed decision-making, ensuring that strategies are built on solid ground.
Optimal Use of Resources:
- Overview: Automation is about doing more with less, ensuring that both human and technological resources are utilized optimally.
- Impact: By taking care of routine and repetitive tasks, automation empowers teams to shift their attention to innovation and other value-driven tasks. This not only boosts employee morale by engaging them in meaningful work but also drives forward-thinking initiatives that can redefine the trajectory of the business.
All told, data automation enables data teams to deliver their data 10x faster at half the cost.
Sarwat Fatima, Principal Data Engineer at Biome Analytics, shares a data automation use case and benefits tailored for the healthcare sector based on her extensive experience.