Updated November 14, 2024
Are you dealing with a scattered data environment, with complex pipelines that seem to go everywhere and nowhere at the same time? Is your data stuck in separate areas within your company, making it hard to use effectively?
Here's the deal: for data to truly drive your business forward, you need a reliable and scalable system to keep it moving without hiccups. In other words, you need data orchestration.
In this article, we'll break down what data orchestration is, its significance, and how it differs from data pipeline orchestration. Yes, they may seem similar, but they are indeed distinct concepts. Adding 'pipeline' into the discussion shifts the focus, presenting a unique set of considerations. We'll also explore how a cutting-edge data orchestration framework can streamline your company's workflows, enabling faster and better-informed business decisions.
What Is Data Orchestration?
Data orchestration is the process of efficiently coordinating the movement and processing of data across multiple, disparate systems and services within a company. This includes but is not limited to, job process sequencing, metadata synchronization, cataloging of data processing results, and managing the interdependencies of various data tasks.
When you really dig into the data orchestration definition, it comes down to a strategy to get all your different data elements — sources, infrastructure, and management practices — to work together into a cohesive and manageable workflow. The goal is to minimize manual intervention and maximize the efficiency of data use.
In this context, the orchestration of data spans a wider range of activities, focusing on the overall coordination of data tasks across the organization. This contrasts with data pipeline orchestration, which adopts a narrower focus, centering on the construction, operation, and management of data pipelines. Data pipeline orchestration is characterized by a detailed understanding of pipeline events and processes. In comparison, general data orchestration does not offer this degree of contextual insight
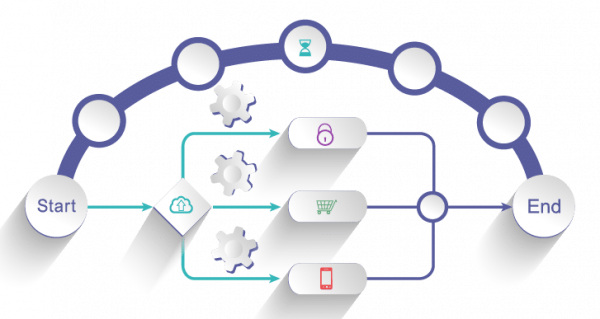
Read More: What Is Data Pipeline Orchestration and Why You Need It
Why Data Orchestration Is Important (But an Unnecessary Complication?)
Not every team needs data orchestration. When data is infrequently updated or accessed, it's possible to utilize raw data directly to fulfill business objectives, provided that cost and performance metrics are met.
However, this approach quickly shows its limitations as data volume escalates. When data starts piling up from all corners — including cloud APIs, cloud warehouses, on-premises databases, and data lakes — that's when you really start feeling the need for efficient data orchestration.
So, why is data orchestration a big deal? Let's break it down:
- Efficiency and Scalability: Data orchestration streamlines the management of complex data workflows, enabling businesses to efficiently handle large volumes of data from diverse sources. It automates and optimizes data processes, reducing manual effort and the likelihood of errors.
- Data Quality and Reliability: By ensuring that data tasks are executed in the correct order and that dependencies are properly managed, data orchestration contributes to higher data integrity and reliability. This is crucial for making informed business decisions based on accurate and up-to-date information.
- Agility and Adaptability: As businesses grow and evolve, their data needs change. Data orchestration provides the flexibility to adapt data workflows — integrating new data sources or modifying existing processes to meet emerging business requirements.
But let's step back for a second.
While recognizing the importance of data orchestration, it's crucial to understand that its increasing necessity has been driven by two distinct trends in the data space:
- The Modern Data Stack: Our data pipelines often consist of a patchwork of different solutions. Each component of the data stack is designed to address specific issues, but this can lead to fragmented workflows. Data orchestration evolved as a means to integrate and coordinate these disparate elements, ensuring a more seamless flow of data across the entire stack.
- Custom-Built (Bespoke) Data Platforms: Another key driver behind the rise of data orchestration has been the trend of data teams creating their own bespoke platforms. These custom-built solutions are tailored to specific organizational needs but can result in highly complex systems. Data orchestration provides a framework to manage these complexities, ensuring that custom workflows and integrations function effectively and efficiently.
Despite its importance, an alternative approach to data orchestration exists. If data pipelines are constructed end-to-end within a single, integrated platform that inherently offers orchestration capabilities, the need for a separate orchestration layer may be reduced or even eliminated.
In such an environment, orchestration is not a standalone task but an integrated feature of the system, potentially simplifying data management and reducing the reliance on multiple specialized tools or an extra layer.
Something to think about, right? But in this post, we're sticking with the idea that you still might need a standalone orchestration tool. Let's dive into how this works and how you can make the most of it.
Read More: How to Build a Data Pipeline in 6 Steps
How Data Orchestration Works
Data orchestration basically acts as that layer that effectively consolidates data-related tasks into a single, end-to-end process. Here is how data orchestration actually works.
The Key Components
- Integration of Disparate Data Sources: First off, data orchestration begins by bringing together various data sources. Whether it's cloud-based systems, on-premises databases, or data lakes, orchestration platforms are designed to connect these dots seamlessly.
- Workflow Creation and Management: Once the data sources are integrated, the next step is setting up workflows. These are essentially the blueprints that define how data moves and transforms across systems. It involves specifying the sequence of tasks, from extraction and transformation to loading and analysis.
- Automating and Optimizing Processes: Data orchestration takes repetitive, manual tasks off your plate. Think of it as setting up a series of dominoes; once you tip the first one, everything else follows in a precise, pre-defined pattern. This automation not only saves time but also reduces the chance of errors creeping in.
- Monitoring and Error Handling: It's not just about setting things in motion. Data orchestration also involves keeping an eye on the whole process. This includes monitoring the performance, spotting any bottlenecks or errors, and jumping in to fix them.
- Adaptability and Scalability: Lastly, data orchestration isn't set in stone. It's built to adapt. As your data needs grow or change, your orchestration setup can scale and morph to fit those new requirements.
Bringing It All Together
So, how does all this come together? Imagine you've got data streaming in from different sources: sales figures from one system, customer feedback from another, and market trends from yet another. Data orchestration takes all these pieces, processes them according to your predefined workflows, and ensures that the resulting insights are ready for you to make those crucial business decisions.
This is the essence of how data orchestration operates. It's a blend of integration, automation, monitoring, and adaptability, all working together to make your data processes more efficient and your life a bit easier.
Here's how a basic orchestration process unfolds in practice:
- Design: Define the data workflows, including sources, destinations, transformations, and conditions.
- Develop: Implement the workflows using orchestration tools, scripting languages, or both.
- Deploy: Set the workflows to run in a production environment with the necessary resources.
- Operate: Monitor the system, manage exceptions, and ensure that the dataflow is smooth and error-free.
- Optimize: Continuously improve the workflows for efficiency, cost, and performance.
Read More: End-to-End Data Pipelines: Hitting Home Runs in Data Strategy
Implementing Data Orchestration: Key Considerations
Before we jump into the key consideration for data orchestration implementation, let's be clear: this isn't a detailed, step-by-step playbook. Think of it more as a checklist of what to keep in mind when you're ready to bring data orchestration into your organization. It's about making smart choices and setting yourself up for success.
1. Assess Your Current Data Ecosystem:
Begin by evaluating your current data management practices. What are your data sources and where does all that data need to end up? What formats does your data exist in? Understanding the existing landscape is crucial for determining the scope and requirements of your orchestration solution.
Action Items:
- Map out your data sources and destinations.
- Document how your data flows right now, including any scheduling that's already in place.
2. Define Your Data Orchestration Goals:
Now, what do you really want to get out of data orchestration? Maybe it's about speeding up how quickly you get insights, or it could be making your data workflows smoother. Set these goals clearly — they'll be your North Star as you pick your tools and map out your strategy.
Action Items:
- Set measurable goals for your data orchestration efforts.
- Consult with stakeholders to make sure everyone's on the same page.
3. Choose the Right Data Orchestration Tool:
This part is crucial. Pick a tool that not only fits your goals but also plays nice with the tech you already use. Think about scalability, user-friendliness, and how well it can handle complex workflows.
Action Items:
- Do your homework on different tools and see which ones fit your needs.
- Try out a few top choices in a pilot project to see them in action.
4. Plan for Scalability and Complexity:
Your tool of choice should not only handle your needs today but also be ready to grow with you. Look for tools that can handle an increasing number of systems, workflows, and datasets without performance degradation.
Action Items:
- Assess the scalability features of data orchestration tools.
- Ensure that the tool can handle the projected increase in data volume and complexity.
5. Design for Fault Tolerance and Recovery:
Things go wrong sometimes. Data orchestration tools should be resilient, with built-in mechanisms to handle failures. Implementing retries, alerts, and fallback strategies will minimize disruptions to data workflows.
Action Items:
- Make sure the tool supports error handling and retry logic in your workflows.
- Set up alerting mechanisms for immediate notification of failures.
6. Avoid Common Mistakes:
Common pitfalls in data orchestration include underestimating the complexity of data workflows, neglecting error handling, and failing to document processes. Staying ahead of these will save you a lot of trouble later.
Action Items:
- Review and document each data workflow in detail.
- Regularly revisit and test error handling procedures.
- Maintain up-to-date documentation for your orchestration processes.
By following these key considerations, you'll lay a strong foundation for data orchestration that's robust, scalable, and in line with what your business needs. Remember, the goal is to create a seamless dataflow that drives insights and value, not to simply move data from point A to B.
Read More: Data Pipeline Automation: The What, How, and Why
Embracing Automation: The Future of Data Orchestration
Remember how we touched on the idea of an end-to-end data pipeline tool that might make standalone orchestration unnecessary? Well, here's a bit more on what that looks like.
Tools that encompass the data pipelines end-to-end are engineered to alleviate the burdens that once fell heavily on data teams — navigating complex conditional branches, adhering to rigid schedules, and handling manual reruns are becoming tasks of the past.
But here's the revolutionary part: these platforms aren't just about providing an all-in-one solution for data pipeline management and orchestration. They're pushing the boundaries into the realm of comprehensive data automation. Orchestration is a critical component, sure, but it's just the starting point in the journey.
These advanced automation platforms enable a more dynamic approach, where adaptive scheduling and intelligent, self-healing systems are the norm. They are designed to anticipate the needs of data workflows, adapting to changes in real-time and ensuring data moves not just with precision, but with an anticipatory understanding of the business context.
Sounds exciting, doesn't it? It's like stepping into a new era of data management where complexity is simplified, efficiency is the standard, and automation is the key to unlocking your data's full potential. If you're ready to take a look and explore these possibilities for your data strategy, just let us know.