This article is based on a presentation given by Paul DeSalvo, Data Engineer Manager at Brazen, at the Data Pipeline Automation Summit 2023. The information presented here is a summary of his insights and experiences about his startup data team.Reading not your thing? Dive right into Paul's full presentation at the Data Pipeline Automation Summit 2023.
When launching a startup, one of the most critical decisions involves choosing the right data tools and technologies to power your business operations. Startups often grapple with several organizational and technical constraints, making the decision of whether to build or buy a challenging one.In this article, we explore these challenges in depth:
- The significant organizational and technical constraints startups face, dissecting the 'build vs buy' dilemma in the context of these limitations.
- Drawing upon real-world examples, we share how Brazen uses Ascend to overcome these constraints and maximize efficiency.
- We provide actionable insights and offer a roadmap for startups navigating the intricate landscape of technology decisions.
Company and Technical Constraints for Startup Data Teams
In the fast-paced world of startups, managing organizational and technical constraints is a constant juggling act. It becomes critical to be adept at navigating these challenges to achieve growth.
Startup Company Constraints
A lean startup environment presents a trio of primary organizational constraints:
- Tight Budgets: In a startup setting, the scarcity of financial resources leads to a high degree of scrutiny for every expenditure. Each dollar spent must yield maximum value and every investment has an opportunity cost. Funding one project often means that another project goes underfunded.
- Lean Staff: For many startups, there is no dedicated team for data projects. At best, there might be one dedicated individual or a handful of team members able to contribute fractions of their time. The lack of human resources adds complexity to the initiation and execution of data projects.
- Competing Priorities: In the rapid-fire environment of a startup, priorities shift quickly. Team members could be called to address an urgent customer issue, or to push a new initiative. Projects evolve, timelines change, and this fast-paced environment can make it difficult to kickstart and maintain momentum for data projects.
Navigating these constraints can sometimes mean that a data project doesn't even take off. But even if you clear this hurdle and gain some resources, a new set of challenges emerges: the technical constraints.
Technical Constraints
Technical constraints pose another significant hurdle for data projects in a startup environment. These constraints include:
- Large Datasets: Startups generate a significant volume of data, from product features to log data. The availability of cheap storage makes it easy to stockpile this information. However, a sudden increase in customers can lead to exponential growth in data, which may outpace the capability of traditional analysis tools.
- Data from Multiple Sources: As a startup grows, it typically starts to use a range of third-party tools for different business functions, each generating its own data. This leads to complex business questions that span multiple data sources, complicating data management and analysis.
- No Single Source of Truth: The challenge of multiple data sources is compounded by the lack of a unified data repository. Analyzing data from disparate sources often means exporting it into spreadsheets, where maintaining consistency and up-to-dateness becomes a Herculean task.
- Inconsistent Calculations: With multiple analyses taking place across different spreadsheets, inconsistencies are likely to creep in. The lack of a single source of truth leads to a situation where the validity of the data is frequently called into question.
In essence, the burden of managing large datasets, coupled with the absence of a single source of truth, forces startups into a cycle of data compromise. They aggregate large datasets into smaller ones, extract CSVs from various third-party applications, and often rely on manual tools like Excel or Google Sheets to bring it all together. Each new analysis becomes a separate endeavor, adding to the overhead and complicating data operations.The critical question then arises: how can startups scale and enhance the efficiency of their data management in the face of these constraints? The answer lies in their choice between building their own data management solutions or buying from external vendors.
Read More: The Hidden Challenges of the Modern Data Stack
The Build vs Buy Dilemma
When it comes to building a data platform at a startup, the 'build vs buy' decision is a critical crossroads. The choice between creating a solution internally or purchasing an off-the-shelf product carries several important considerations, each with its own merits and drawbacks. Here are the key considerations:
Cost
One of the most immediate considerations in this equation is cost. At first glance, building might seem like the more cost-effective route. Many startups already have some basic infrastructure in place that can be further developed, and the perceived savings compared to the high price tag of an enterprise solution can be appealing. However, these initial savings can be deceptive.While an in-house solution may serve current needs and appear low-cost initially, as data grows exponentially, what worked for a small data set may no longer be effective. Scaling becomes an issue, potentially exploding the budget. This problem is further compounded by the increased maintenance needs of an evolving system and the potential lack of in-house expertise to manage the growing complexities. As such, the true cost of building might be significantly higher than initially expected, particularly if these challenges slow down your speed to market and transform the project into a cost center instead of a revenue generator.
Customization
Building your solution can offer customization potential, allowing you to tailor your tools to your specific use case. However, this aspect should be weighed against the fact that a built solution may not scale well or may require constant tweaking and maintenance as your startup grows and changes.
Time to Market
In contrast, buying a solution can offer significant advantages in time to market. A faster implementation timeline means getting data products in front of customers sooner. If the product can generate revenue swiftly, the initial investment can be recouped quickly, and the project can even start contributing positively to the company's bottom line. This revenue can also fuel further resource allocation to the project, improving its potential for success.
Expertise
Purchasing a solution also brings the added benefit of the vendor's expertise. Most providers in the data management field have years of experience and dedicated teams to handle a wide range of data challenges. With a purchased solution, your startup can leverage this expertise without having to spend time and resources developing it in-house. This advantage not only adds value to the solution but also frees up your team to focus on your startup's core business goals.
Maintenance
When it comes to maintaining your data management system, buying offers distinct advantages. Providers of off-the-shelf solutions often have dedicated maintenance teams to ensure that their products continue to perform optimally. This maintenance, which might include updates, bug fixes, and system improvements, is generally included in the purchase price. This offloads the burden of maintaining a complex data management system from your startup's team, enabling them to focus on more strategic tasks.
Scalability
Scalability is another important consideration in the build vs buy debate. When you buy a solution, you're usually purchasing a product that has already been proven to scale. Vendors often build their products to accommodate a wide range of business sizes and data volumes, with the expectation of company growth. This stands in contrast to building a solution in-house, which might work for the present volume of data but could struggle to keep pace with the rapid growth common to successful startups.
Integration
Finally, integration is an important benefit of purchasing a solution. Vendors often provide a range of integration options with their products, knowing that their solutions need to fit within a larger ecosystem of tools and systems. These integrations can save considerable time and effort that would otherwise be spent trying to connect disparate systems. When you purchase a solution, you're not just buying a product—you're buying a piece of a puzzle designed to fit seamlessly into your business operations.
Read More: From Patchwork to Platform: The Rise of the Post-Modern Data Stack
Brazen's Use Case: How Ascend Maximizes Efficiency
As a startup data team, Brazen found itself grappling with these very questions when determining how to handle its data engineering needs.
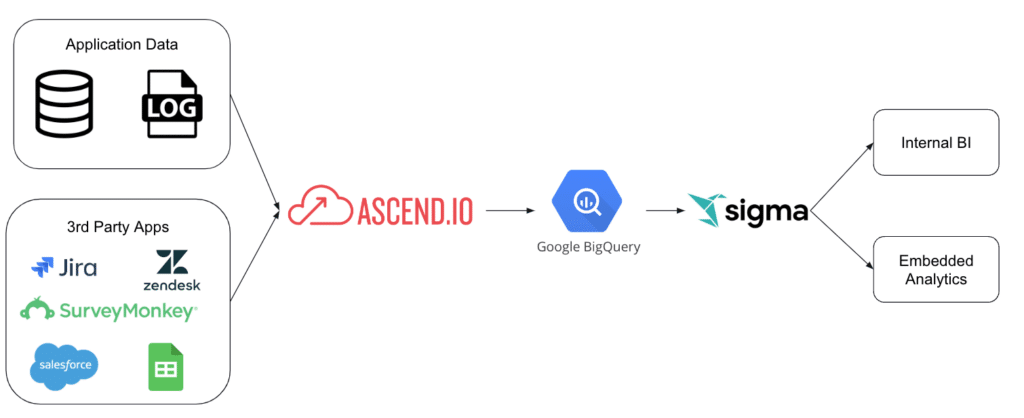
The Challenge
Brazen is a lean tech startup in the HR tech space. Their data engineering team consists of just one person. Brazen needed a solution that allowed for rapid data ingestion, transformation, and sharing. They needed to answer business questions quickly, providing same-day responses to data inquiries. The choice of vendor could make or break their project, determining whether it remained stuck in 'onboarding hell' or moved forward to deliver value.
The Ascend Solution
Ascend offered a flexible, scalable solution that catered to Brazen's specific needs. It allowed Brazen to connect data sources quickly and start deriving value immediately.
- Speed and Agility: With Ascend, Brazen can access new data from their databases within minutes, vastly improving their response time to business questions.
- The Learning Curve: Brazen recognized that while Ascend came with a learning curve, mastering advanced functionalities like data ingestion and partitioning ultimately offered more flexibility. With Ascend's help, Brazen found the onboarding process not just fruitful but also engaging.
- Data Variety: Ascend's ability to handle a wide range of data types and sources proved invaluable. By defining transformations and schemas on data as it's ingested, Ascend ensures data consistency and quality.
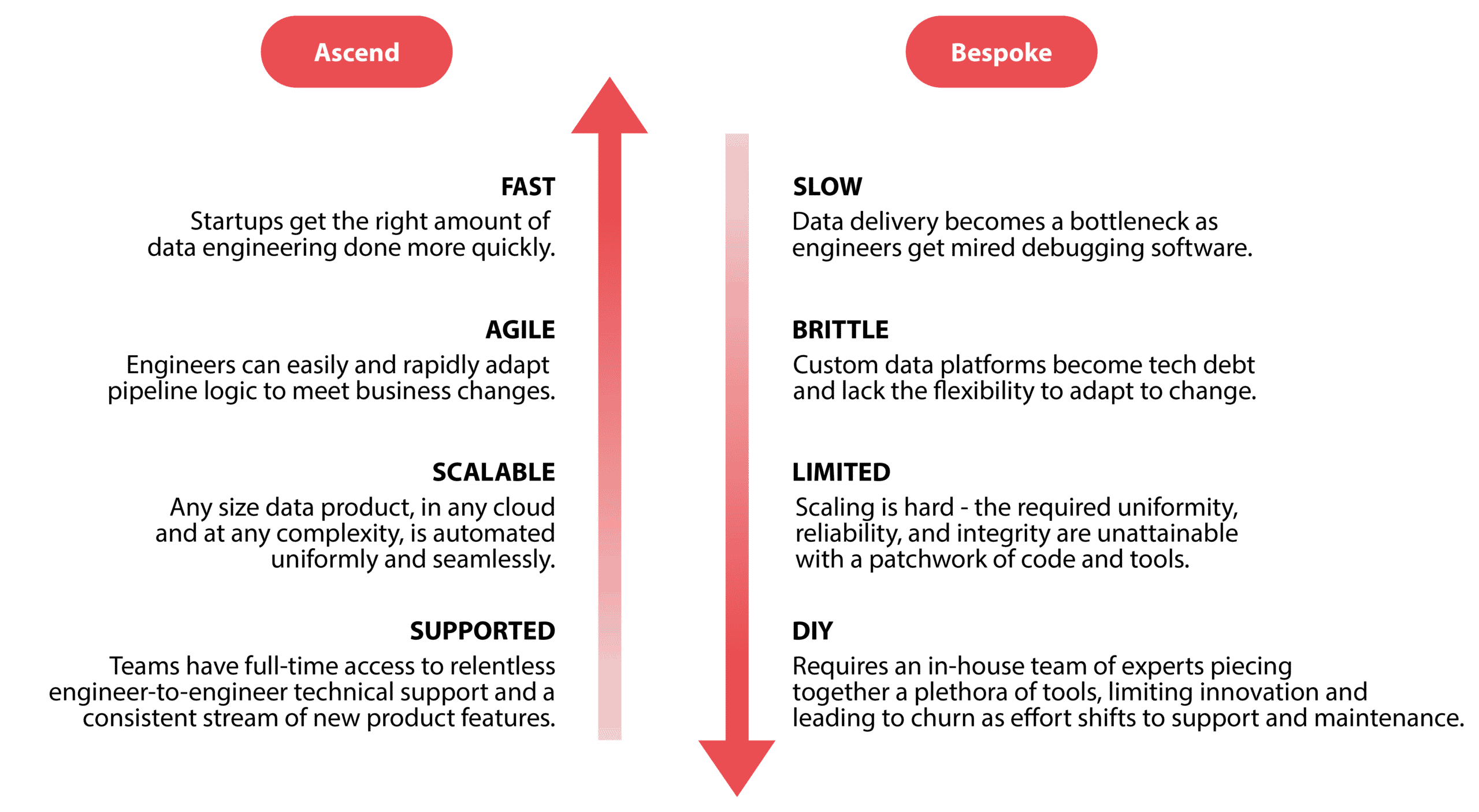
The Outcome
By choosing to buy instead of building, Brazen's startup data team managed to avoid 'reinventing the wheel' and focused its energy on activities that bring direct value to its customers. Using Ascend, Brazen has managed to stay nimble, answering data inquiries quickly, and continuously delivering value to their users.
Read More: Winning the Startup Race with Data Automation
Ascend's Role in Startup Success
In the build vs buy paradigm, the decision ultimately rests on understanding your startup's unique constraints and needs. As Brazen's experience shows, buying from a trusted vendor like Ascend can empower startups to overcome both organizational and technical constraints, enabling them to focus on delivering customer value and achieving business success.Ascend's robust data solutions enabled Brazen to transition from being data-overwhelmed to being data-driven. In doing so, Brazen truly embodied its name, boldly and effectively navigating the competitive business terrain. This underscores the idea that with the right tools and partners, startups can overcome limitations and actualize their potential.
Read More: What Is Data Pipeline Automation?